Graph RAG: The Future of Intelligent Retrieval
Aug 22, 2024
Aug 22, 2024
Aug 22, 2024
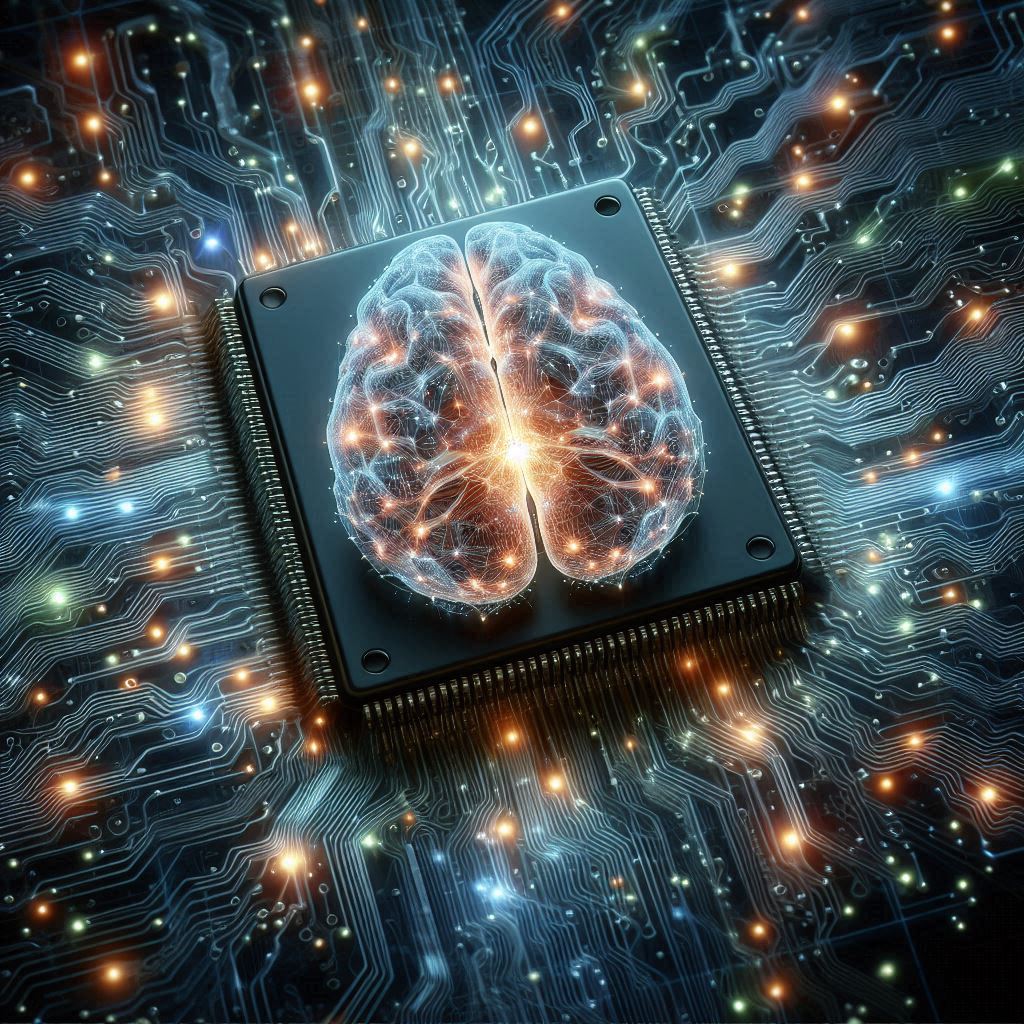
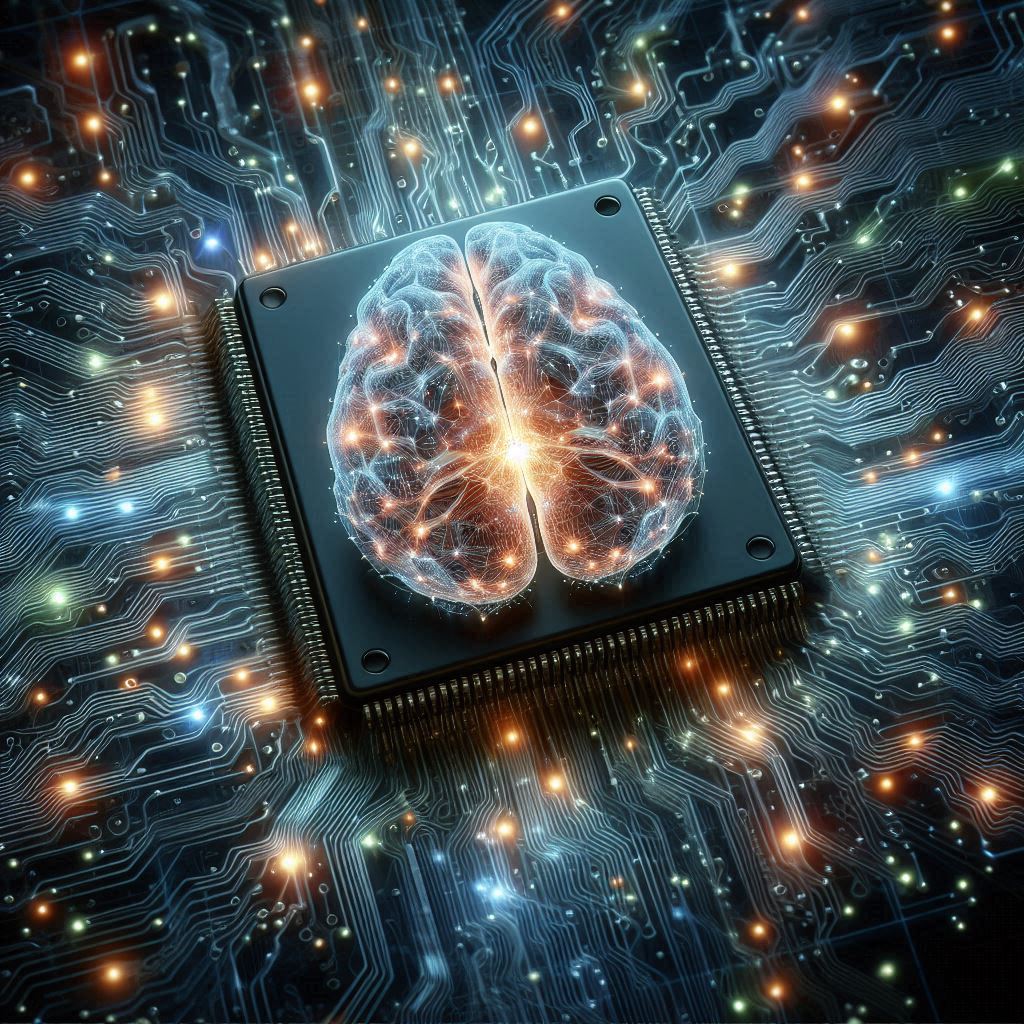
In a world where AI is evolving faster than your last software update, staying ahead of the curve isn't just about keeping up—it's about innovating. And when it comes to making the most out of your data, there's a new player in town: Graph RAG. Whether you're a tech enthusiast or a decision-maker looking to harness the full potential of AI, Graph RAG is the next big thing you need to know about.
What’s the Deal with RAG?
Before diving into Graph RAG, let's talk about RAG—Retrieval-Augmented Generation. This is where AI searches through your data to find the most relevant bits and then uses that information to generate answers. Think of it as a librarian who not only finds the books you need but also gives you a detailed summary.
But here’s the kicker: while traditional RAG does a good job, it sometimes misses the mark, especially when it needs to connect complex dots or understand deep semantic concepts. Enter Graph RAG, the superhero sidekick to your AI, ready to take retrieval to the next level.
Why Settle for Text When You Can Have a Graph?
Imagine trying to solve a jigsaw puzzle with just a few scattered pieces. That’s what traditional RAG sometimes feels like. Graph RAG, however, gives you the whole picture—complete with connections between entities that traditional methods might overlook.
Graph RAG doesn’t just extract chunks of text; it uses a knowledge graph—a structured web of information that shows how entities are connected. This isn’t just about making sense of the data; it’s about giving your AI the ability to think in a more human-like way.
Three Flavors of Graph RAG: Pick Your Favorite
Graph RAG isn't one-size-fits-all. Depending on your needs, you can customize how you use it:
1. Graph as a Content Store: Want to dig deep into documents and come up with the most relevant information? This variety uses a knowledge graph to extract document chunks, combining it with metadata for a richer search experience. It’s like having a super-powered search engine tailored to your needs.
2. Graph as a Subject Matter Expert: Need to understand complex concepts? This version extracts relevant entities and their relationships, providing your AI with a semantic context. It’s like giving your AI a PhD in your domain.
3. Graph as a Database: Want to turn natural language questions into precise graph queries? This version maps your questions to graph queries, runs them, and then asks the AI to summarize. It's the perfect blend of precision and depth.
Why Should You Care?
Sure, this all sounds impressive, but what does it mean for you? Here’s the bottom line: if you're dealing with large datasets, complex information, or the need for deep insights, Graph RAG can be a game-changer.
For the tech-savvy: Imagine having an AI that not only understands your data but also connects the dots like a seasoned detective. For decision-makers: Picture making choices based on AI insights that are more accurate and contextually rich, giving you the edge in a competitive market.
Overcoming Challenges:
Because Every Superhero Has Their Kryptonite
Of course, building and maintaining a knowledge graph isn’t a walk in the park. It requires expertise, ongoing maintenance, and thoughtful design. But the rewards? They’re worth it.
- Scalability and Performance: As your knowledge graph grows, ensuring efficient storage and querying can be challenging. But with the right strategies, these hurdles can be overcome, ensuring smooth performance even at scale.
- Data Integration: Bringing together data from different sources? No problem. Graph RAG can help you create a cohesive, integrated knowledge base that powers your AI like never before.
- Transparency: In a world where AI decisions need to be explainable, knowledge graphs provide a transparent way to see how conclusions are reached, building trust and clarity.
The Future Is Here: Small Graphs and Multi-Agent Systems
What’s next for Graph RAG? Imagine smaller, more specialized graphs working in tandem—each managed by a different agent, each contributing to a larger understanding. This approach isn’t just the future; it’s the next evolution in making AI smarter, faster, and more intuitive.
Final Thoughts: Don’t Just Keep Up—Lead
Graph RAG is more than just a buzzword; it’s a powerful tool that can transform how you interact with data. Whether you’re deep in the tech trenches or making strategic decisions, understanding and implementing Graph RAG could be the key to staying ahead in the AI race.
So, are you ready to unleash the full potential of your data? With Graph RAG, the future of intelligent retrieval is not just within reach—it’s here.
Sources
In a world where AI is evolving faster than your last software update, staying ahead of the curve isn't just about keeping up—it's about innovating. And when it comes to making the most out of your data, there's a new player in town: Graph RAG. Whether you're a tech enthusiast or a decision-maker looking to harness the full potential of AI, Graph RAG is the next big thing you need to know about.
What’s the Deal with RAG?
Before diving into Graph RAG, let's talk about RAG—Retrieval-Augmented Generation. This is where AI searches through your data to find the most relevant bits and then uses that information to generate answers. Think of it as a librarian who not only finds the books you need but also gives you a detailed summary.
But here’s the kicker: while traditional RAG does a good job, it sometimes misses the mark, especially when it needs to connect complex dots or understand deep semantic concepts. Enter Graph RAG, the superhero sidekick to your AI, ready to take retrieval to the next level.
Why Settle for Text When You Can Have a Graph?
Imagine trying to solve a jigsaw puzzle with just a few scattered pieces. That’s what traditional RAG sometimes feels like. Graph RAG, however, gives you the whole picture—complete with connections between entities that traditional methods might overlook.
Graph RAG doesn’t just extract chunks of text; it uses a knowledge graph—a structured web of information that shows how entities are connected. This isn’t just about making sense of the data; it’s about giving your AI the ability to think in a more human-like way.
Three Flavors of Graph RAG: Pick Your Favorite
Graph RAG isn't one-size-fits-all. Depending on your needs, you can customize how you use it:
1. Graph as a Content Store: Want to dig deep into documents and come up with the most relevant information? This variety uses a knowledge graph to extract document chunks, combining it with metadata for a richer search experience. It’s like having a super-powered search engine tailored to your needs.
2. Graph as a Subject Matter Expert: Need to understand complex concepts? This version extracts relevant entities and their relationships, providing your AI with a semantic context. It’s like giving your AI a PhD in your domain.
3. Graph as a Database: Want to turn natural language questions into precise graph queries? This version maps your questions to graph queries, runs them, and then asks the AI to summarize. It's the perfect blend of precision and depth.
Why Should You Care?
Sure, this all sounds impressive, but what does it mean for you? Here’s the bottom line: if you're dealing with large datasets, complex information, or the need for deep insights, Graph RAG can be a game-changer.
For the tech-savvy: Imagine having an AI that not only understands your data but also connects the dots like a seasoned detective. For decision-makers: Picture making choices based on AI insights that are more accurate and contextually rich, giving you the edge in a competitive market.
Overcoming Challenges:
Because Every Superhero Has Their Kryptonite
Of course, building and maintaining a knowledge graph isn’t a walk in the park. It requires expertise, ongoing maintenance, and thoughtful design. But the rewards? They’re worth it.
- Scalability and Performance: As your knowledge graph grows, ensuring efficient storage and querying can be challenging. But with the right strategies, these hurdles can be overcome, ensuring smooth performance even at scale.
- Data Integration: Bringing together data from different sources? No problem. Graph RAG can help you create a cohesive, integrated knowledge base that powers your AI like never before.
- Transparency: In a world where AI decisions need to be explainable, knowledge graphs provide a transparent way to see how conclusions are reached, building trust and clarity.
The Future Is Here: Small Graphs and Multi-Agent Systems
What’s next for Graph RAG? Imagine smaller, more specialized graphs working in tandem—each managed by a different agent, each contributing to a larger understanding. This approach isn’t just the future; it’s the next evolution in making AI smarter, faster, and more intuitive.
Final Thoughts: Don’t Just Keep Up—Lead
Graph RAG is more than just a buzzword; it’s a powerful tool that can transform how you interact with data. Whether you’re deep in the tech trenches or making strategic decisions, understanding and implementing Graph RAG could be the key to staying ahead in the AI race.
So, are you ready to unleash the full potential of your data? With Graph RAG, the future of intelligent retrieval is not just within reach—it’s here.
Sources