From RAG to Riches - Unveiling the working of Retrieval-Augmented Generation
Jul 15, 2024
Jul 15, 2024
Jul 15, 2024
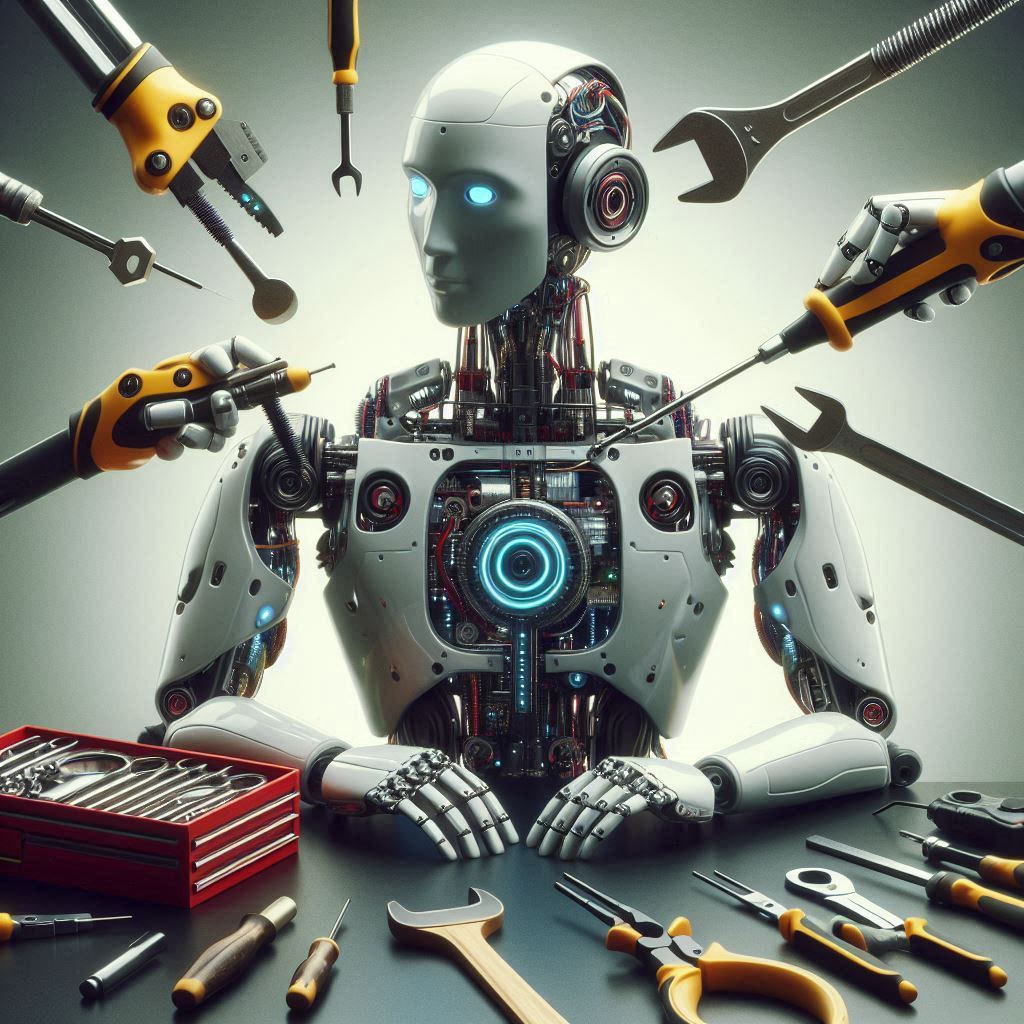
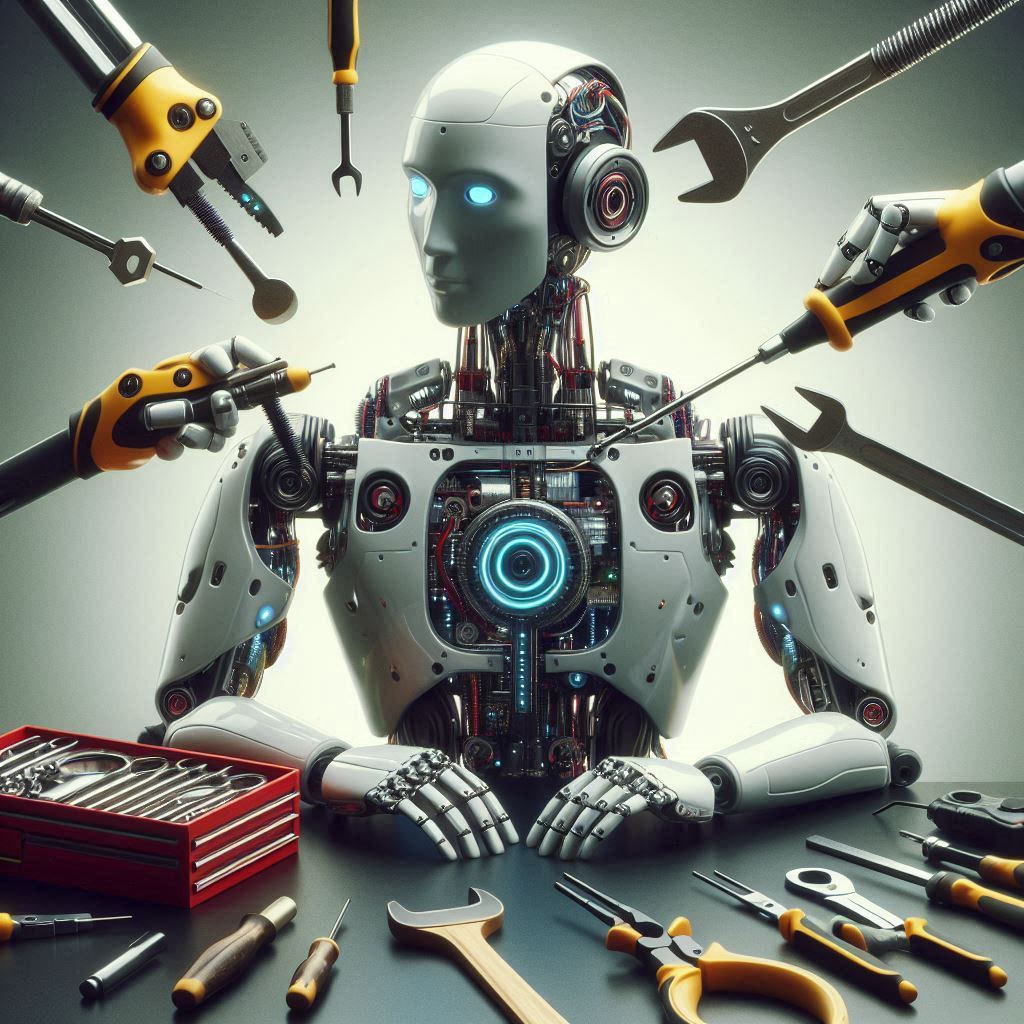
Level Up Your AI
Remember those limitations of Large Language Models (LLMs) we talked about last time? The whole "stuck-in-a-textbook" syndrome? Yeah, not ideal for real-world applications.
But fear not, AI enthusiasts, because there's a superhero in town – Retrieval-Augmented Generation, also known as RAG!
In this sequel to our LLM lowdown, we're diving deep into RAG's superpowers. Think of it as giving your AI a real-time research assistant to fact-check and supercharge its responses.
Ready to unlock the true potential of your AI? Let's explore the inner workings of RAG!
How Does RAG Work?
With RAG, we introduce an information retrieval component that fetches new, relevant data based on the user’s query. This fresh information is then combined with the LLM's training data to create a more accurate response.
Meet the Generator: Weaving the Answer’s Tapestry
The Retriever collects all the relevant documents (databases, documents, news feeds) which can be used to answer the user query. It sources data from all over the internet and comes up with a bunch of stuff that might help the LLM generate a response.
With the top relevant document retrieved, it’s now the Generator who produces a final answer by synthesizing and expressing that information in natural language.
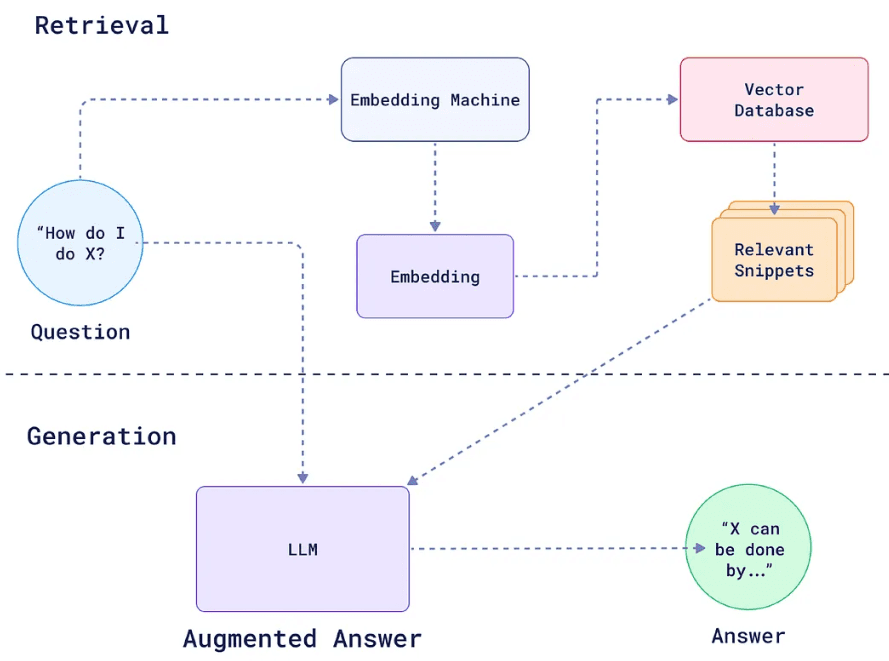
What in the heaven is the “Embedding Machine” and “Embedding” in the diagram ?
RAG's Secret Weapon: How Indexing Makes AI Sharper
A indexing system lets RAG find the most relevant information quickly, just like finding the right book in a well-organized library.
Here's how it works:
From Docs to Digits: RAG takes all the documents it needs and converts them into a special code called an "index."
Question Transformation: Your question also gets a makeover! RAG converts it into its own "index," focusing on the keywords and meaning.
Similarity Search: RAG compares the question's index to the document indexes in its library. The documents with the most similar indexes are the likely suspects for the answer.
The Perfect Match: RAG picks the documents that best match the question and feeds their information to the LLM
The more accurate the indexing, the better RAG can understand your questions and deliver insightful responses.
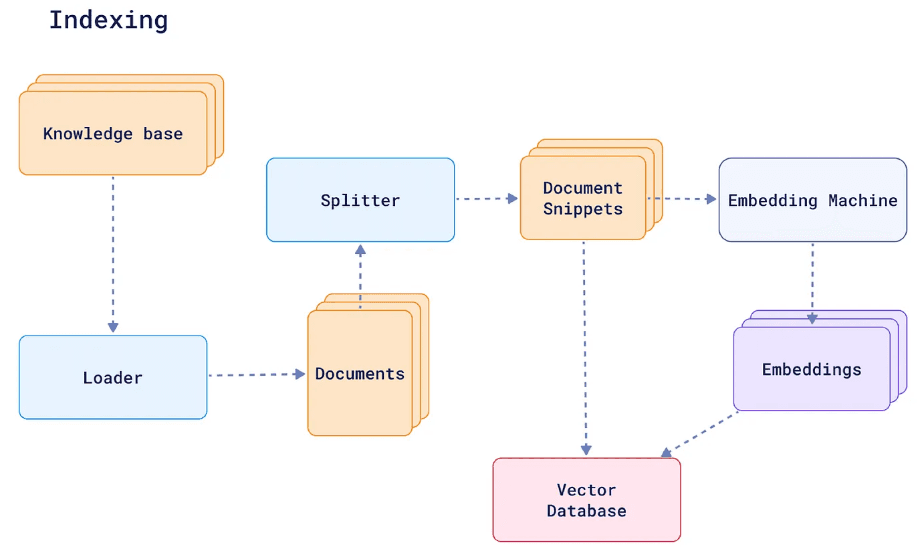
The flow begins…
The system follows a series of steps to retrieve and generate responses.
You Ask, RAG Listens: You fire off a question or request to your AI.
Retrieval Gets Real: RAG's retrieval system kicks in, searching external sources like databases or news feeds for relevant information.
Matching Mania: RAG sifts through the information, looking for the best matches to your question based on keywords and context.
Knowledge Boost: RAG feeds the most relevant information to the AI's generative model.
AI in Action: The generative model uses its knowledge and the info from RAG to craft a response that's both creative and accurate.
The user input is used twice, once to fetch relevant resources from the user and another time to actually perform the search on the fetched chunk of data.
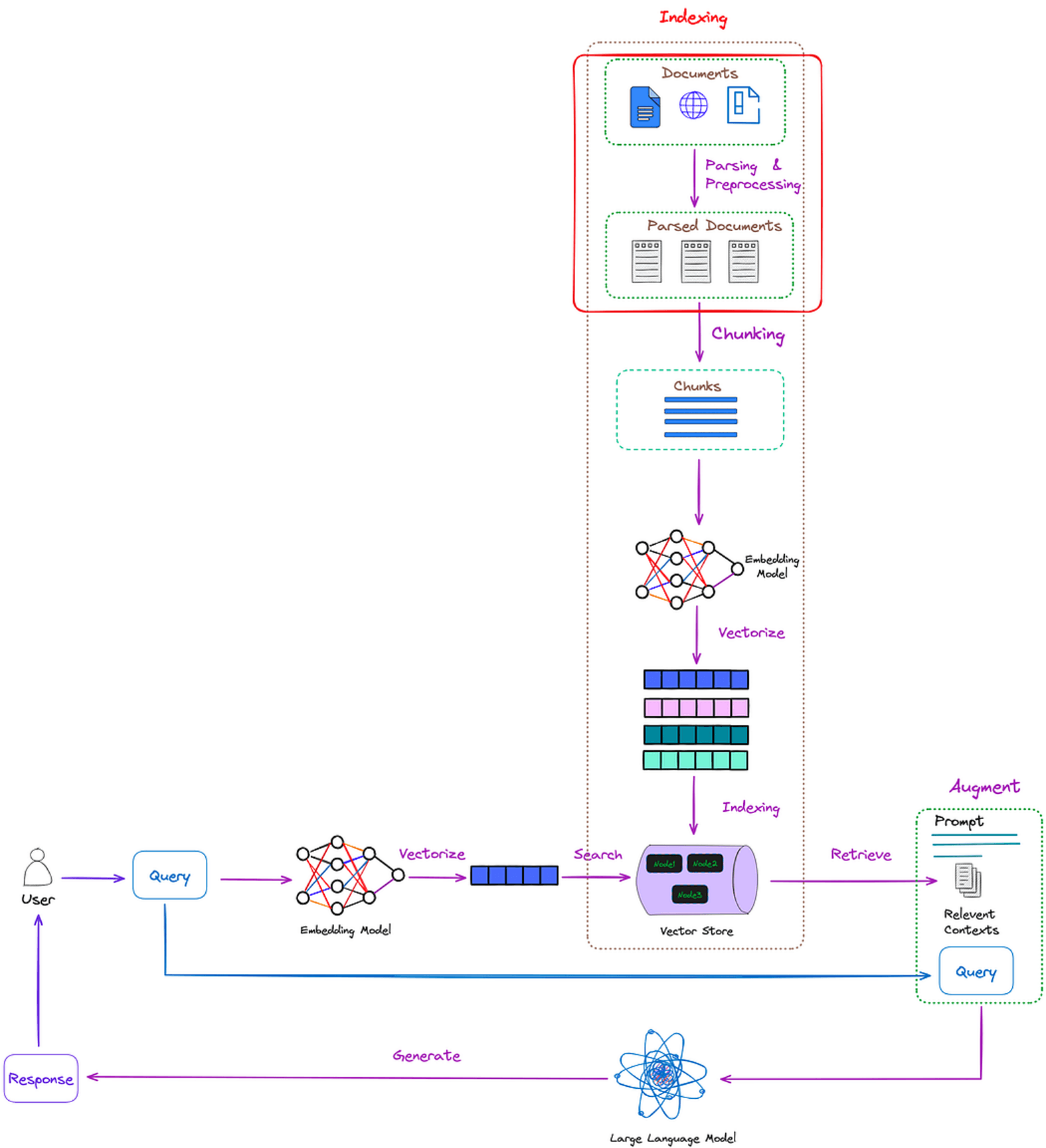
Use Cases: Transforming Everyday Business Needs
RAG technology isn't just a novelty; it's a transformative tool for everyday business challenges. Here are some compelling use cases illustrating how RAG can revolutionize your operations:
Customer Support Chatbots
Imagine a customer support chatbot that provides accurate, timely responses by pulling the latest data from your knowledge base. RAG ensures customers receive current and relevant information, enhancing satisfaction and reducing the support team's workload.
Example: A telecom company uses a RAG-powered chatbot to answer inquiries about the latest plans, technical issues, and real-time service updates, resulting in happier customers and a more efficient support system.
Internal Knowledge Management
RAG streamlines information retrieval within large organizations, helping employees find relevant documents, policies, and procedures quickly. This boosts productivity and ensures access to accurate information.
Example: An HR department uses RAG to provide employees with up-to-date details on leave policies, benefits, and company protocols, eliminating the need to search through multiple documents.
Personalized Marketing and Sales
RAG enhances marketing and sales efforts by offering personalized responses and recommendations based on real-time data. This tailors communication to individual customer needs and preferences.
Example: An e-commerce company uses RAG to generate personalized product recommendations for shoppers, leading to higher conversion rates and increased customer loyalty.
Conclusion
The potential applications of RAG in business are vast and varied. RAG can significantly improve the accuracy, efficiency, and effectiveness of your operations. Don't miss out on the opportunity to revolutionize your business with RAG technology.
Ready to see the difference RAG can make? Contact us to discover how RAG can be seamlessly integrated into your business solutions and drive your operations to new heights!
Sources
Level Up Your AI
Remember those limitations of Large Language Models (LLMs) we talked about last time? The whole "stuck-in-a-textbook" syndrome? Yeah, not ideal for real-world applications.
But fear not, AI enthusiasts, because there's a superhero in town – Retrieval-Augmented Generation, also known as RAG!
In this sequel to our LLM lowdown, we're diving deep into RAG's superpowers. Think of it as giving your AI a real-time research assistant to fact-check and supercharge its responses.
Ready to unlock the true potential of your AI? Let's explore the inner workings of RAG!
How Does RAG Work?
With RAG, we introduce an information retrieval component that fetches new, relevant data based on the user’s query. This fresh information is then combined with the LLM's training data to create a more accurate response.
Meet the Generator: Weaving the Answer’s Tapestry
The Retriever collects all the relevant documents (databases, documents, news feeds) which can be used to answer the user query. It sources data from all over the internet and comes up with a bunch of stuff that might help the LLM generate a response.
With the top relevant document retrieved, it’s now the Generator who produces a final answer by synthesizing and expressing that information in natural language.
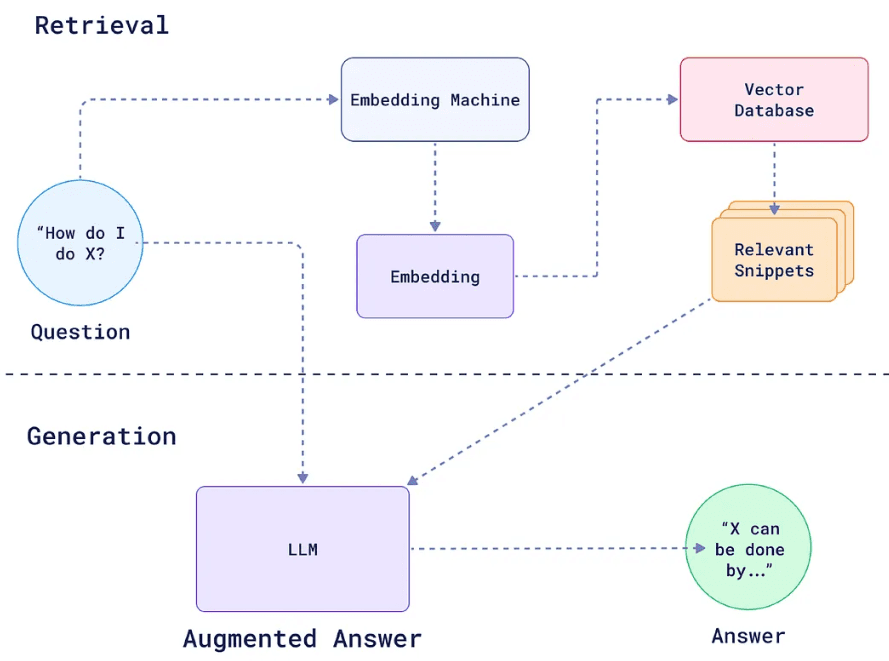
What in the heaven is the “Embedding Machine” and “Embedding” in the diagram ?
RAG's Secret Weapon: How Indexing Makes AI Sharper
A indexing system lets RAG find the most relevant information quickly, just like finding the right book in a well-organized library.
Here's how it works:
From Docs to Digits: RAG takes all the documents it needs and converts them into a special code called an "index."
Question Transformation: Your question also gets a makeover! RAG converts it into its own "index," focusing on the keywords and meaning.
Similarity Search: RAG compares the question's index to the document indexes in its library. The documents with the most similar indexes are the likely suspects for the answer.
The Perfect Match: RAG picks the documents that best match the question and feeds their information to the LLM
The more accurate the indexing, the better RAG can understand your questions and deliver insightful responses.
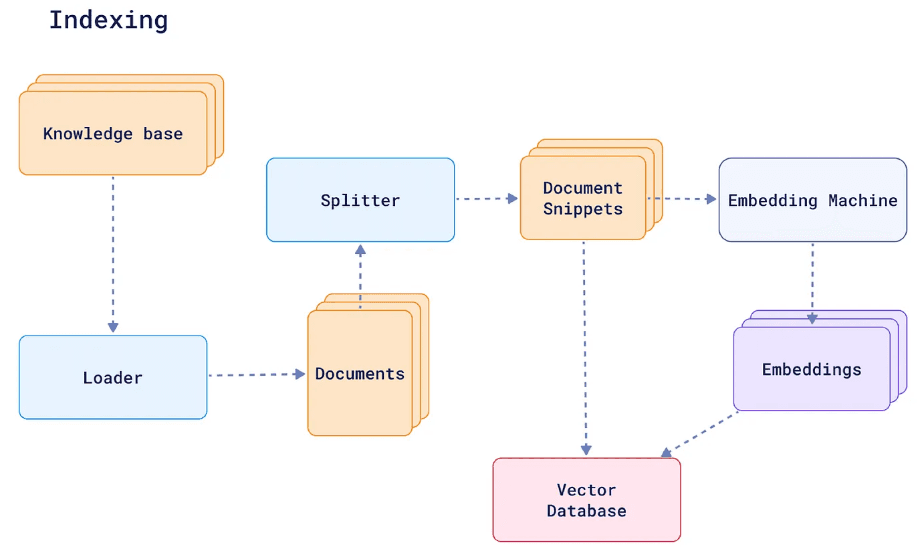
The flow begins…
The system follows a series of steps to retrieve and generate responses.
You Ask, RAG Listens: You fire off a question or request to your AI.
Retrieval Gets Real: RAG's retrieval system kicks in, searching external sources like databases or news feeds for relevant information.
Matching Mania: RAG sifts through the information, looking for the best matches to your question based on keywords and context.
Knowledge Boost: RAG feeds the most relevant information to the AI's generative model.
AI in Action: The generative model uses its knowledge and the info from RAG to craft a response that's both creative and accurate.
The user input is used twice, once to fetch relevant resources from the user and another time to actually perform the search on the fetched chunk of data.
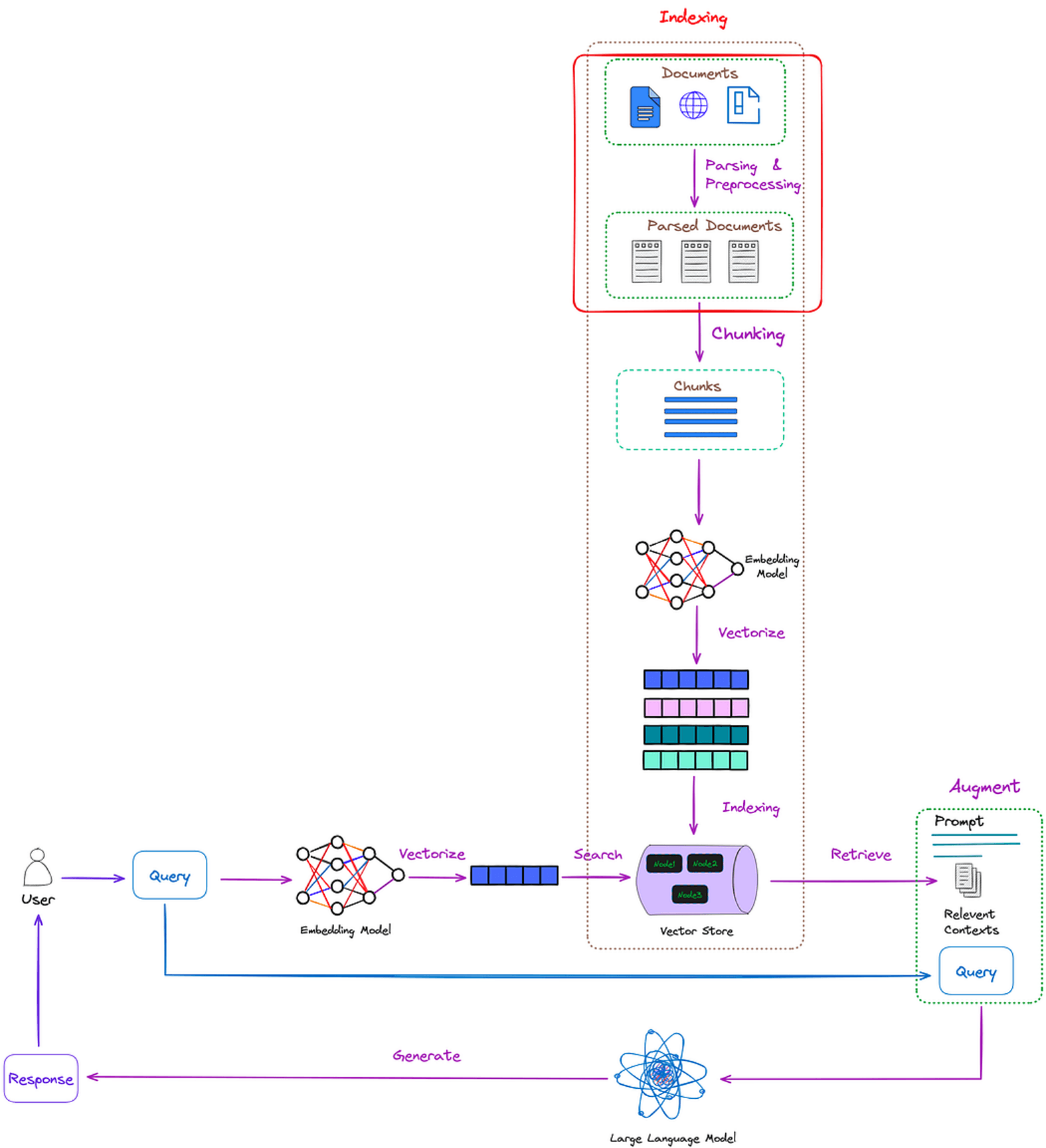
Use Cases: Transforming Everyday Business Needs
RAG technology isn't just a novelty; it's a transformative tool for everyday business challenges. Here are some compelling use cases illustrating how RAG can revolutionize your operations:
Customer Support Chatbots
Imagine a customer support chatbot that provides accurate, timely responses by pulling the latest data from your knowledge base. RAG ensures customers receive current and relevant information, enhancing satisfaction and reducing the support team's workload.
Example: A telecom company uses a RAG-powered chatbot to answer inquiries about the latest plans, technical issues, and real-time service updates, resulting in happier customers and a more efficient support system.
Internal Knowledge Management
RAG streamlines information retrieval within large organizations, helping employees find relevant documents, policies, and procedures quickly. This boosts productivity and ensures access to accurate information.
Example: An HR department uses RAG to provide employees with up-to-date details on leave policies, benefits, and company protocols, eliminating the need to search through multiple documents.
Personalized Marketing and Sales
RAG enhances marketing and sales efforts by offering personalized responses and recommendations based on real-time data. This tailors communication to individual customer needs and preferences.
Example: An e-commerce company uses RAG to generate personalized product recommendations for shoppers, leading to higher conversion rates and increased customer loyalty.
Conclusion
The potential applications of RAG in business are vast and varied. RAG can significantly improve the accuracy, efficiency, and effectiveness of your operations. Don't miss out on the opportunity to revolutionize your business with RAG technology.
Ready to see the difference RAG can make? Contact us to discover how RAG can be seamlessly integrated into your business solutions and drive your operations to new heights!
Sources